Innovations
Pushing the boundaries of AI technology and research
Whitepapers
Open frameworks. Practical insights. Ethical AI built to scale.
Tailored Intelligence for Industry-Specific Performance
Custom LLMs Engineered for Enterprise Precision
General-purpose models are powerful — but they're not enough.
In complex enterprise environments, success depends on accuracy, compliance, and deep domain alignment. That's why at ExpertOps AI, we build Custom LLMs designed for your specific industry, workflows, and business rules.
These models don't just understand language — they understand your language.
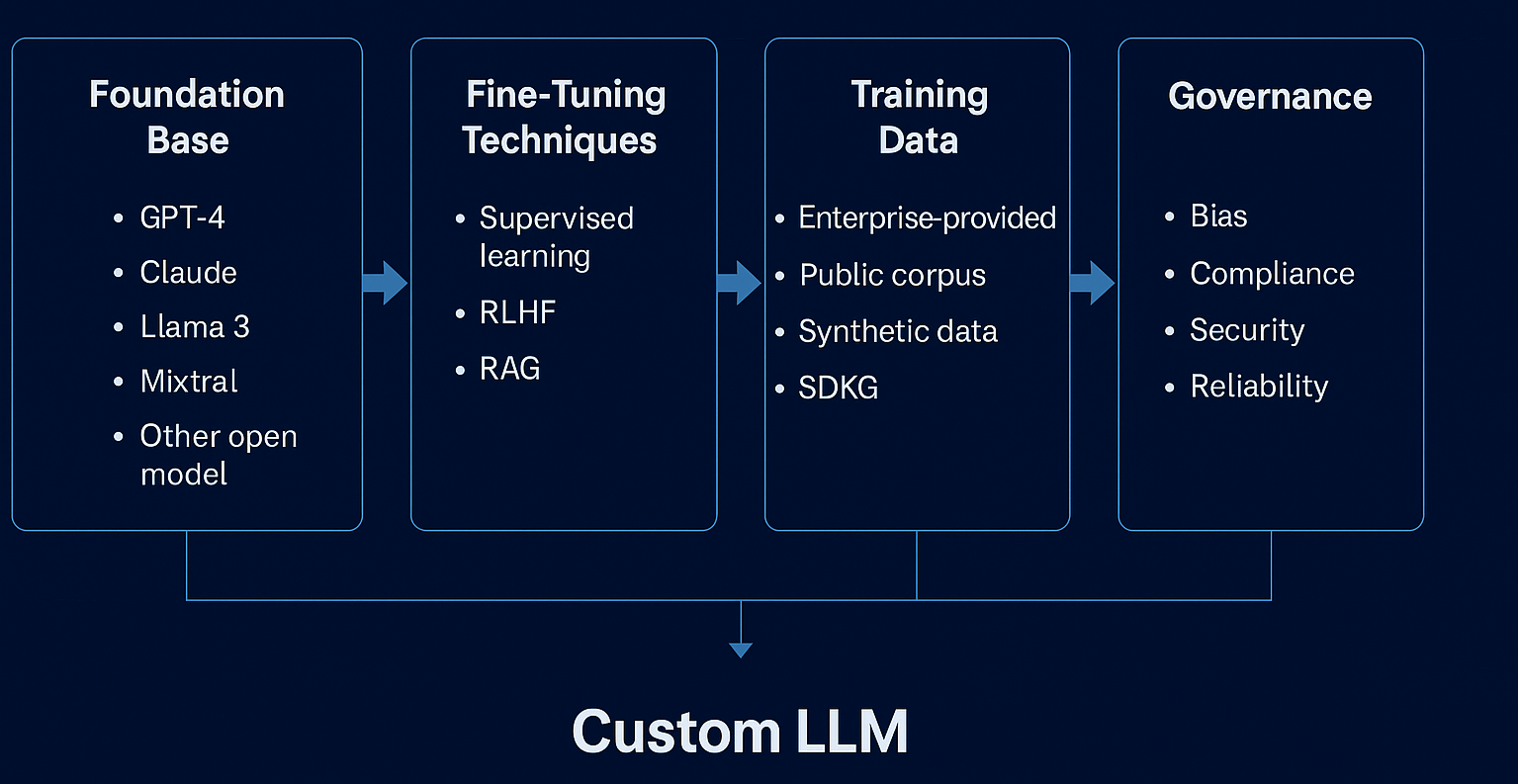
🔧 How We Build & Deploy Custom Models
- Foundation Models: GPT-4, Claude, Llama 3, Mixtral, or a model of your choice
- Techniques: Supervised learning, domain-specific RLHF, Retrieval-Augmented Generation (RAG), and adaptive fine-tuning
- Data Sources: Your enterprise data, public corpora, synthetic datasets, and SDKG (Synthetic Domain Knowledge Generation)
- Governance: Every model is rigorously tested for bias, compliance, safety, and performance.
Looking to build your own? We support collaborative fine-tuning and secure on-prem deployment.
Open Source Contributions
Built on Open Innovation. Growing with the Community.
At ExpertOps AI, we believe in the power of open-source — not just as a foundation, but as a force for progress. Many of the technologies we build upon, extend, or integrate with are open-source, and we're proud to contribute back to the ecosystem that fuels AI innovation.
Whether it's fine-tuning workflows, data pipelines, agent orchestration, or enterprise governance layers, our open-source work reflects our commitment to transparency, scalability, and community impact.
Have an idea, integration, or improvement you'd like to contribute? Want to work with us on a new open-source tool? We'd love to hear from you.
Responsible AI
Trust Built In. Governance by Design.
At ExpertOps AI, we believe that powerful AI must also be principled. That's why our platform is built with governance, transparency, and safety embedded at every layer — from model orchestration to agent behavior.
Responsible AI at ExpertOps AI is not a feature — it's a foundational standard. Whether you're in healthcare, finance, aviation, or government, your digital employees are governed, monitored, and aligned to your values and compliance needs.
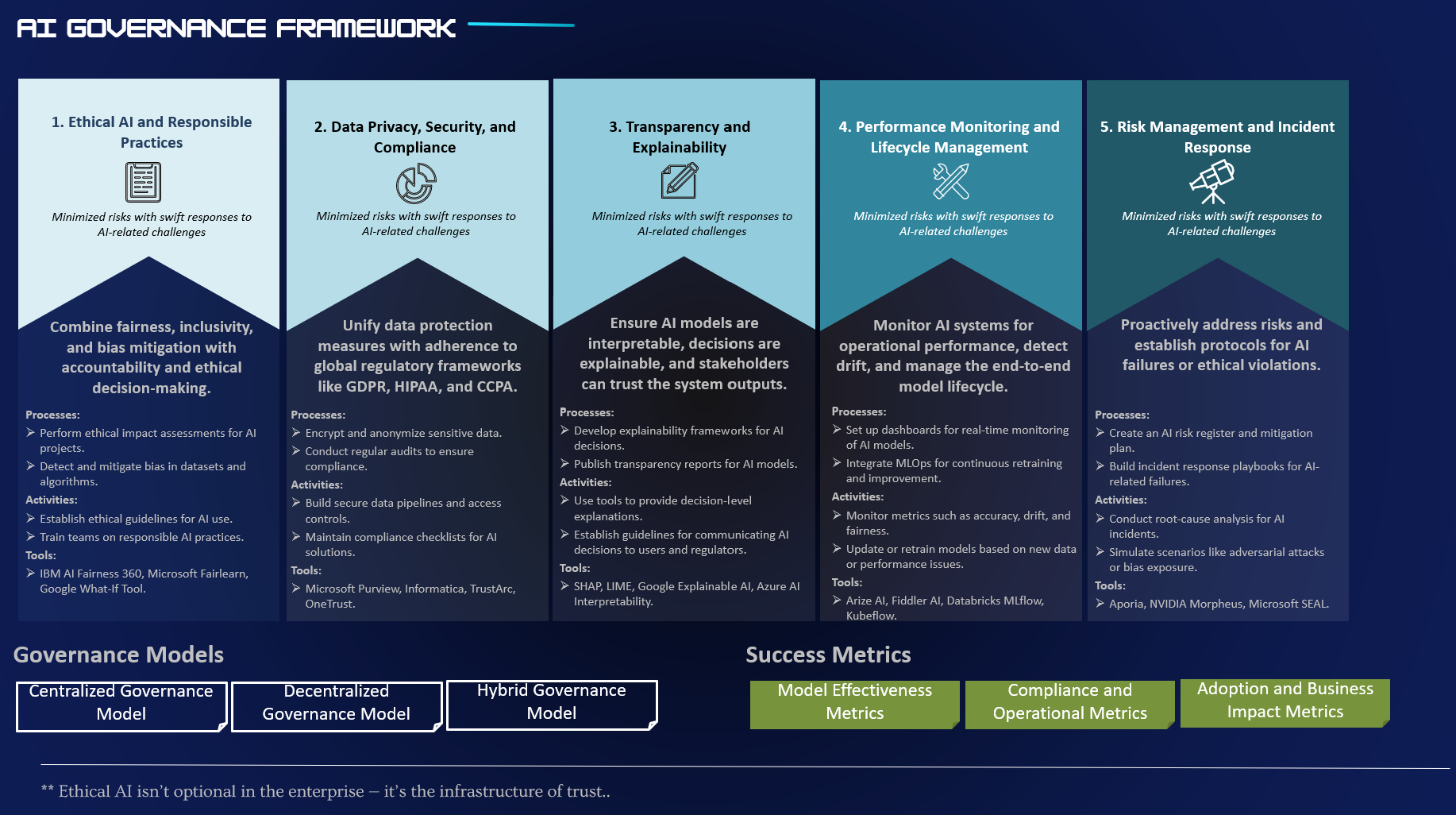
Core Principles of Responsible AI at ExpertOps AI
- Explainability & Transparency
Agents generate audit trails and rationale for decisions — so you can trace, verify, and validate every action taken. - Human-in-the-Loop Oversight
You stay in control. Insert human approvals or interventions where required — especially for high-stakes workflows. - Fairness & Bias Mitigation
We monitor model behavior for fairness and ensure AI outputs are tested against domain-specific bias risks. - Privacy & Security by Design
All data interactions are governed by role-based access, encryption, and your compliance protocols (GDPR, HIPAA, SOC2, etc.). - Governance Controls at Scale
Enterprise policies are embedded directly into agent behavior — including usage restrictions, escalation paths, and logging. - Lifecycle Management
Deployed agents are continuously monitored, retrained, or retired to maintain accuracy, performance, and relevance.
Ethical AI isn't optional in the enterprise — it's the infrastructure of trust.
At ExpertOps AI, we make Responsible AI operational, scalable, and real.